The rise of Large Language Models (LLMs) and Language-Agnostic Models (LAMs) has reshaped AI, yet their distinct applications are often overshadowed by hype. LLMs are celebrated for their deep understanding of language nuances, while LAMs pioneer adaptability across various languages without being tied to any specific one. Understanding these models’ unique capabilities and limitations can guide software developers and business leaders toward informed choices.
As companies integrate AI, balancing expectations with technical capabilities becomes crucial. LLMs and LAMs each offer strengths suited to specific tasks. LLMs excel in linguistic richness, suited to single-language processing, while LAMs bring flexibility for diverse languages, avoiding reliance on monolingual datasets. This article uncovers often-overlooked factors, providing insights into each model’s true potential and guiding readers on when to leverage LLMs or LAMs for efficiency and precision. Learn more about the risks of marketing hype around AI models.
Core Differences between LLMs and LAMs
The Technology Behind LLMs and LAMs
LLMs and LAMs differ in architecture, purpose, and functionality. LLMs rely on deep learning and are optimized for generating nuanced language, adapting well to specific contexts and subtleties in languages like English or Spanish. They focus on a language-specific dataset and excel in tasks requiring in-depth linguistic understanding. In contrast, LAMs use language-agnostic datasets, making them capable of cross-linguistic functionality without leaning on any single language’s structure. LAMs prioritize content’s universal aspects and are suited to contexts where translation or multilingual understanding is paramount.
From a technical standpoint, LLMs leverage transformer architectures with large-scale datasets refined for specific languages, whereas LAMs employ more versatile methods, often simplifying certain elements to ensure adaptability across multiple languages. This distinction is fundamental when selecting an AI model, as LLMs generally demand more computational power due to their depth, while LAMs sacrifice some depth for broader utility.
Language Flexibility vs. Language Focus: Which Suits Your Needs?
In global applications, choosing between language-specific precision and universal adaptability is critical. LLMs, designed for high-depth language generation, are ideal in monolingual environments where nuanced comprehension matters. For instance, customer service in a single language can leverage LLMs to achieve conversational accuracy.
However, for multilingual businesses, LAMs’ language-agnostic approach can be invaluable. Unlike LLMs, LAMs are trained without bias towards a single language, making them adaptable to cross-cultural requirements. They’re structured to support several languages equally, a unique advantage when handling tasks that span diverse linguistic backgrounds. For companies aiming to serve a global audience, LAMs offer a scalable, inclusive solution, avoiding the extensive training that monolingual LLMs would require for each language.
Accuracy and Precision: Evaluating Quality of Output
Balancing Precision with Cultural Nuance
Accuracy often depends on understanding a language’s cultural context, a strength of LLMs due to their focused datasets. These models excel in interpreting complex sentences or idiomatic expressions with high accuracy, an essential factor in tasks such as content generation or customer interactions within a single language.
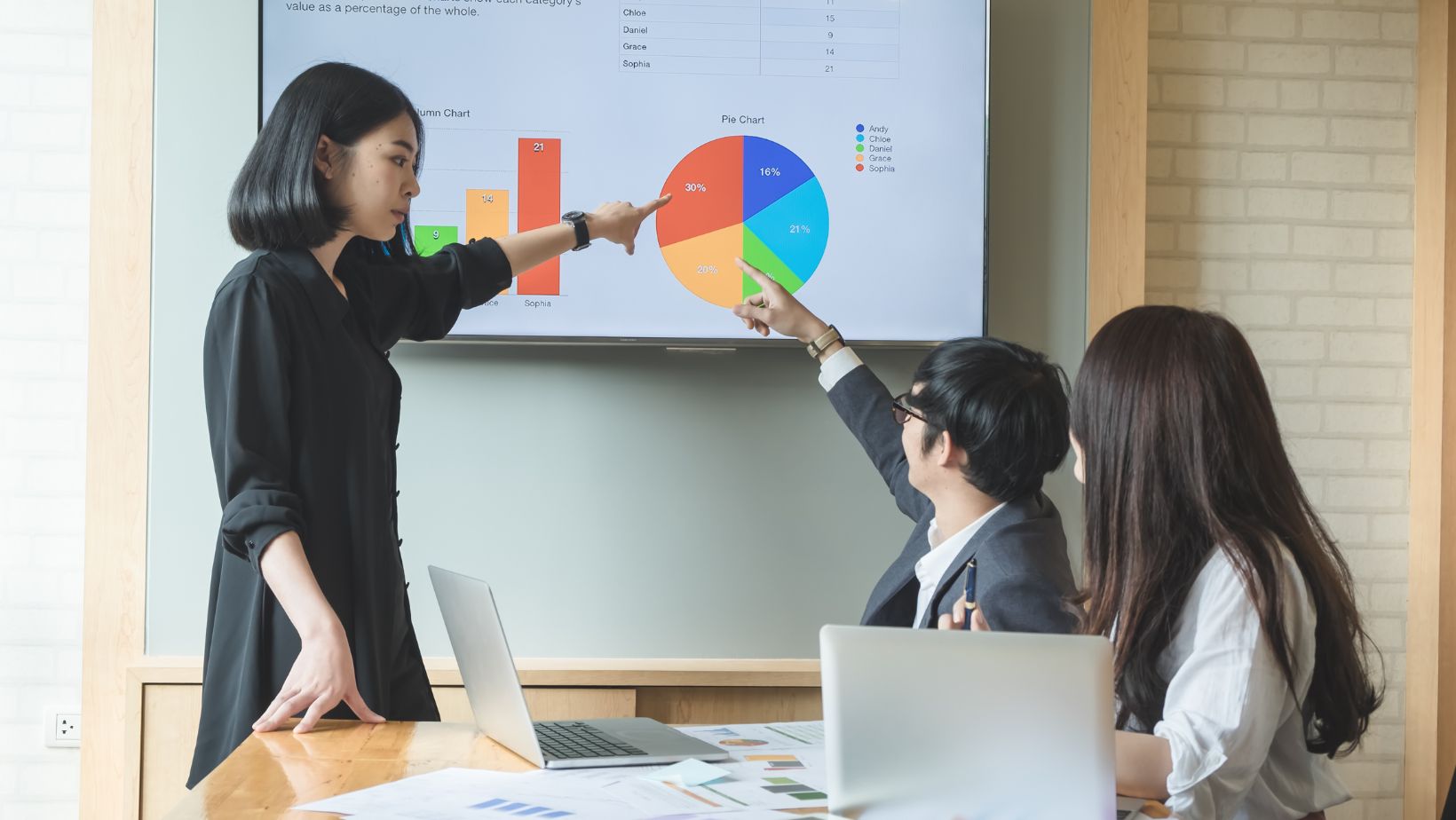
Conversely, LAMs handle linguistic diversity by remaining neutral to language-specific intricacies. They’re engineered for broad understanding, favoring multi-language accuracy over single-language precision. While this can result in less nuanced translations, LAMs ensure consistent quality across languages, a major advantage in cross-cultural applications where exact replication of local linguistic nuances isn’t required. This difference highlights a trade-off between cultural accuracy and linguistic inclusivity, influencing how each model supports business goals.
Resource Efficiency and Scalability
Scalability and resource efficiency are significant considerations in deploying AI models. LLMs, with their intricate models and vast datasets, often demand substantial computational power, limiting scalability for businesses with restricted budgets or processing capabilities. The deep, language-specific training of LLMs results in high memory and energy use, making their deployment cost-intensive for companies focused on long-term scalability.
LAMs, meanwhile, are structured to operate across languages with minimal modification. Their language-neutral design reduces resource strain, requiring less data and processing power per language compared to retraining LLMs. This structural efficiency makes LAMs a more scalable solution, especially for multilingual applications, where a single model can serve multiple markets without requiring extensive customization.
Feature | LLMs | LAMs |
Training Complexity | High, due to language focus | Lower, supports multiple languages |
Resource Intensity | High | Lower |
Scalability | Limited by high resource needs | Scalable across languages |
Ideal Application | Monolingual, language-specific tasks | Multilingual, cross-linguistic tasks |
Cost Implications and ROI of LLMs vs. LAMs
The financial commitment to implementing AI is a central consideration in model selection. LLMs incur higher costs due to their extensive training requirements, specialized datasets, and continuous fine-tuning for precision in specific languages. For businesses prioritizing quality in single-language applications, the investment in LLMs may yield a worthwhile return by improving user satisfaction and engagement.
LAMs, by contrast, offer a cost-effective alternative for companies needing multilingual capabilities without additional training. The shared language model structure of LAMs reduces development and maintenance costs, making them suitable for businesses requiring rapid multilingual scalability. Companies that operate in international markets often see higher ROI from LAMs due to reduced need for language-specific tuning and the ability to deploy quickly across regions.
Ethical and Compliance Factors in Model Deployment
Navigating Cultural and Contextual Bias
Ethics and compliance are vital in model deployment, especially for AI models like LLMs and LAMs that impact diverse audiences. LLMs, trained on language-specific datasets, can inadvertently reflect biases present in those languages or cultures, creating ethical concerns if not properly managed. In customer-facing applications, such biases can compromise fairness, affecting brand reputation and trust.
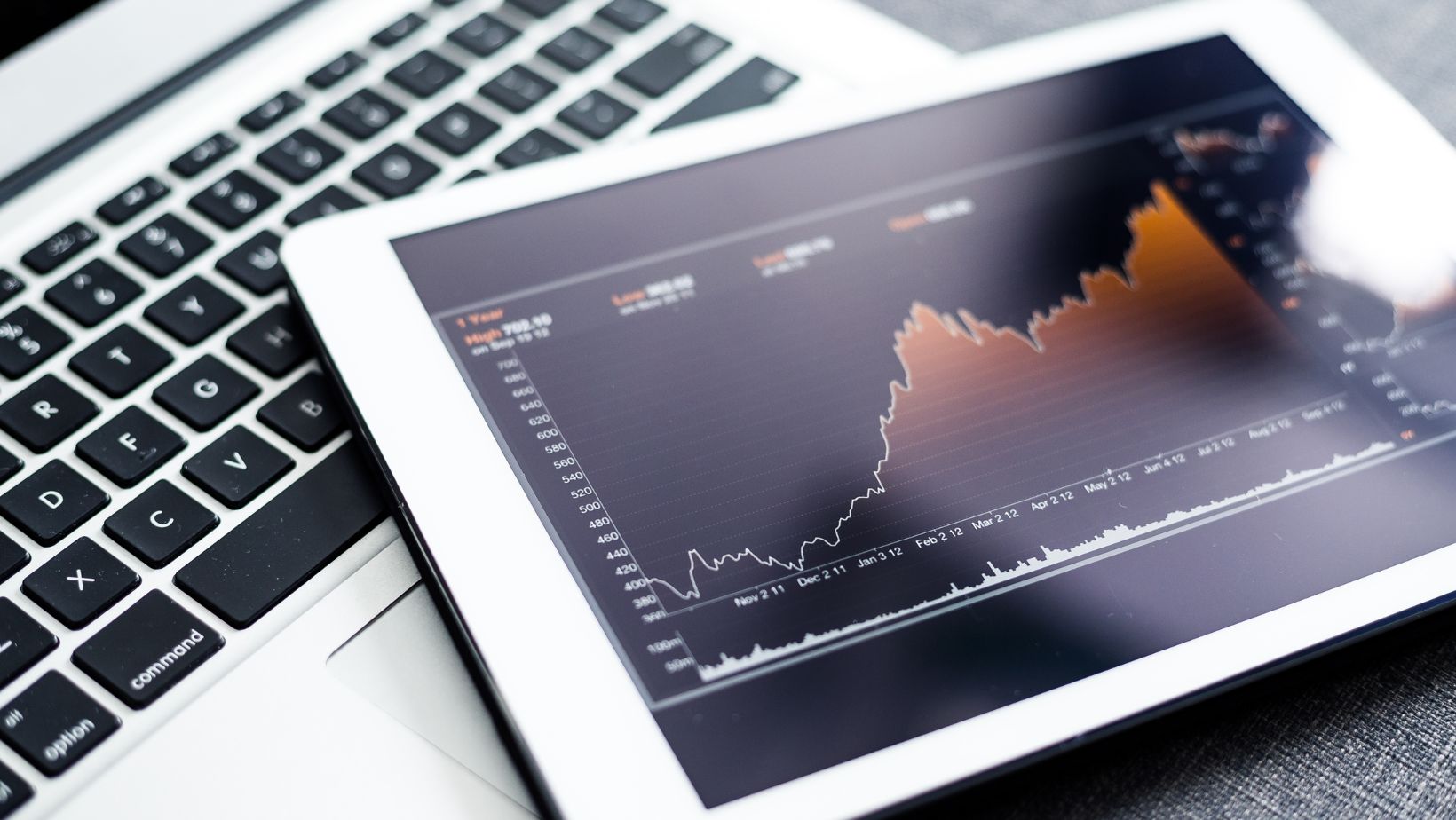
LAMs offer a neutral framework that can help mitigate some cultural biases, as they are designed without favoring any specific language. However, this neutrality can also overlook cultural nuances, making LAMs less suitable where local context and cultural sensitivity are crucial. Businesses need to carefully assess the risk of bias and prioritize ethical training practices for both model types to ensure fair and responsible AI.
Future Outlook: Trends Shaping the Evolution of LLMs and LAMs
As AI advances, both LLMs and LAMs are expected to see new developments that enhance their relevance. The trend toward hybrid models, which combine the nuanced understanding of LLMs with the cross-linguistic flexibility of LAMs, is gaining traction, potentially offering the best of both approaches. These innovations aim to create models that can adapt dynamically, improving both precision and scalability.
Additionally, as computational resources evolve, the cost and resource requirements of LLMs may decrease, enabling broader access to their deep linguistic capabilities. LAMs, meanwhile, continue to develop in areas like multimodal AI, where combining text with images or other data types can enhance understanding across contexts. The future may see both models converging in ways that cater to specific market needs, offering tailored AI solutions that are both accessible and powerful.
Conclusion: Choosing the Right Model for Your Goals
Selecting between LLMs and LAMs involves a nuanced understanding of each model’s benefits and limitations. Companies should weigh factors such as language scope, resource efficiency, and ethical considerations to determine which model aligns with their goals. LLMs offer unparalleled depth in language-specific applications, while LAMs enable cross-linguistic scalability with reduced resource needs. By carefully evaluating each option beyond surface-level promises, businesses can leverage AI in a way that maximizes value and aligns with their long-term vision.